Abstract
This research focuses on problem-based learning (PBL) teaching methods and designs artificial intelligence (AI) in facial recognition systems, smart streetlights, and drone as teaching materials. To integrate teaching materials of life situation issues into the natural general curriculum and develop a learning perception questionnaire (LPQ) with validity and reliability to evaluate students’ perception of the curriculum. Based on a valid assessment tool, 56 college students were assessed on their learning of emerging technology contextual issues to evaluate their satisfaction, learning situation, and learning effectiveness. The results of the study are, as follows:
(1) construct teaching materials for AI application in face recognition systems, street lights, and drone situations; (2) develop an LPQ with reliability and validity; (3) most students are satisfied with the integration of AI into PBL teaching; (4) most students believe that the integration of cross-domain learning in different subjects can help improve self-learning effectiveness and ensure continuous learning interest; and (5) many students agree that this course can improve learning outcomes. In the future, the focus will be on teaching practice, incorporating easy-to-use AI textbook content, and enhancing the opportunities for interactive learning; in addition, increasing the number of effective samples in the research to improve the depth of the experiment and the breadth of research.
License
This is an open access article distributed under the Creative Commons Attribution License which permits unrestricted use, distribution, and reproduction in any medium, provided the original work is properly cited.
Article Type: Research Article
INTERDISCIP J ENV SCI ED, Volume 20, Issue 2, 2024, Article No: e2406
https://doi.org/10.29333/ijese/14420
Publication date: 01 Apr 2024
Online publication date: 27 Mar 2024
Article Views: 2009
Article Downloads: 1038
Open Access HTML Content Download XML References How to cite this articleHTML Content
INTRODUCTION
The 21st century is an era of emerging technological innovations. Industries are emerging rapidly. Artificial intelligence (AI), the Internet of things, augmented reality, virtual reality, and 5G have quietly entered everyone’s lives. In the life circle of AI, the application fields of AI are becoming more diverse, and cross-domain learning combining multiple disciplines is becoming more popular (Rihtaršič et al., 2016; Su, 2021a). The practice of AI includes streetlights, drones, smart cities, self-driving cars, and robots. The applied fields included agriculture, medical care, transportation, financial services, catering, and hotels. Cross-domain and cross-disciplinary learning makes AI a contemporary science. A technological revolutionary tool continues to shine and generate heat.
Nonetheless, in the real world, AI continues to encounter obstacles and restrictions (Benitti, 2012). This problem implements necessary education, additional research development, and learning methods (Sullivan & Heffernan, 2016). Therefore, some advanced countries strive to participate in AI education integration and active research (Alimisis, 2013). Just like the research of Huang (2018), integrating AI into natural science learning is an important learning strategy. This strategy helps to improve students’ learning performance. Problem-based learning (PBL) is a student-centered teaching method (Jansson et al., 2015; Syadiyah et al., 2017), where students learn from their relevant and interesting problem structures. This substantive learning strategy enhances their motivation to learn and promotes the practice of teaching and learning skills such as teamwork, problem-solving, self-discipline, and improved academic performance (Mundilarto, 2018; Syadiyah et al., 2017). PBL has been applied in cross-field teaching and has attracted the attention of scholars (Sakir & Kim, 2020).
The method of PBL is to apply ill-structured problems to acquire new knowledge based on the scientific implementation (Jansson et al., 2015; Syadiyah et al., 2017) and initiate learning problems through life problem-solving, connecting learning experiences and activated learning, and then improving their knowledge. Jansson et al. (2015) proposed PBL teaching method, which could help students improve their problem-solving and assessment learning abilities and deepen their understanding of science courses; Gunter and Alpat (2017) found that PBL achieved significant learning in science learning. Rillero and Chen (2019) also found that PBL can combine different courses to experience meaningful topics. Hernández-Ramos et al. (2021) emphasized that PBL teaching methods provide learning potential for solving practical environmental problems in natural science education.
AI is becoming more and more popular in a variety of fields, including STEM education (Jang et al., 2022), computer science (Di Eugenio et al., 2021), mathematics education (Gadanidis, 2017), and music education (Zulić, 2019), among others, research on its application in various fields shows that AI has the potential to transform and enhance the educational experience. The empirical study on incorporating AI themes into life science scenarios using PBL teaching techniques is fragile, and the breadth of implementation and the number of publications addressing the integration of AI into scientific education remain somewhat limited (Su, 2022).
This study uses PBL teaching method to design three AI life issues to conduct diffusion evaluation research to address this critical gap in the literature. In summary, this AI problem-based life situation strategy teaching guidance and design is an innovation and experience of this teaching method. It aspires to make teaching in the general course “technology society and life” more relevant and students’ learning more vivid and engaging.
Research Purpose & Questions
Based on the above research motivations, this research aims to use PBL teaching method to design three AI issues in facial recognition systems, smart streetlights, and drones in life situations. Teaching materials to assess students’ learning perceptions. Accordingly, the focus issues of this study are, as follows:
-
How to design a life-situation experience teaching material for AI new issues by PBL teaching method?
-
How to develop a valid and reliable learning perception questionnaire (LPQ) to evaluate their satisfaction with course, learning situation, and learning effectiveness?
-
How do students solve the difficulties encountered by their group discussion in cross-disciplinary experience and problem-solving?
LITERATURE REVIEW
Problem-Based Learning
Student-centered PBL, a teaching method based on ill-structure problems, groups for cooperative learning and discussion under the guidance of teachers, analyze and clarify, plan solutions, guide learning directions, find strategies to solve problems based on group discussions, test results and provide feedback analysis, complete PBL process (Jansson et al., 2015; Prince & Felder, 2006; Su, 2022). Jansson et al. (2015) and Yoon et al. (2014) stressed that PBL strategies could help improve problem-solving, evaluate self-learning and skills, and enhance their in-depth learning and profound understanding of the subject content. Gunter and Alpat (2017) studied the application of PBL teaching strategies in science courses and found significant learning effects.
Artificial Intelligence
The difference between AI and human intelligence (HI) is that AI can combine the perception, learning, memory, knowledge, semantics, reasoning, language, and thinking of HI with machine learning to implement it on a computer (Lai, 2016; Su, 2021a). Scholars have found that AI could provide students with personalized teaching and improve problem-solving skills, learning, and decision-making strategic judgments (Ng, 2016; Ricoy & Feliz, 2016). The social applications of AI in life are becoming more diverse, and they are increasingly closely related to human life experience and social survival (Topcu et al., 2010). The implementation of this technology, as Bansal et al. (2016) and Nordhoff et al. (2017) pointed out that driverless cars or buses could enable passengers to focus on business and improve productivity during long-distance travel. Hsu et al. (2020) pointed out the distinction between facial recognition systems and generative adversarial networks that these issues related to scientific cognitive learning literacy and social abilities.
Situated Learning Theory
Situated learning theory emphasizes the presentation of knowledge from the practical application of real-life situations. To combine learning and application, allowing learners to think and practice at the same level as experts and learn through social interaction and collaborative cooperation. Halim and Saat (2017) believe that in the context of this interactive process, students could integrate their relevance to clear expression and make decisions. In addition, they might suitably enhance the range and depth of information learning. However, they apply the knowledge they have gained to communicate and solve problems faced in life and further improve their conceptual understanding and problem-solving abilities (Sevian et al., 2015; Su, 2018). To come true and realize the philosophy that education is life (Su, 2021a).
Problem-solving of AI applications in daily life has become a fundamental issue in the development of emerging technologies. Therefore, this study combines the real-world insights of PBL teaching method with the facial recognition system and smart streetlights of AI life applications to establish situational life teaching materials and effectively develop learning evaluation tools to estimate learners’ learning effectiveness. Figure 1 presents the theoretical framework of learning effectiveness. Figure 1 describes that situated learning and PBL provide students with the ability to think and experience real-world problems, situated learning and AI provide a learning environment for technical practice, and AI and PBL deepen students’ scientific learning literacy. Accordingly, expect the relationship between PBL, AI, and situated learning to improve their learning effectiveness.
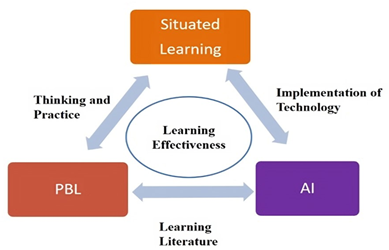
METHODOLOGY
Participants
To study the appropriateness and consistency of the sampling, the sample of this study comes mainly from sophomore students from the school, where the researcher teaches. A total of 56 students from the fourth technical department who took the general course “technology, society and life” participated (28 boys and 28 girls, aged 20-22).
Table 1 presents demographic information about students’ backgrounds and characteristic analyses.
Table 1. Students’ background & characteristic analysis (n=56)
|
Research Design
The students were divided into seven groups according to the homogeneity of their expertise in the department to explore student satisfaction with the course, learning situation, and learning effectiveness evaluation.
Table 2 shows the experimental research design model with two hours per week and a total of five weeks. The teaching experiment process includes an introduction to PBL, application of AI in life, identification of problems, data collection, group discussion construction and presentation of evidence for problem-solving, group presentation, and evaluation of learning contribution. All PBL teaching steps included ill-structured questions, clarifying problems, planning self-directed learning, putting forward problem-solving, reviewing problem-solving, and presenting their final reflection feedback (Lee & Bae, 2008; Su, 2022).
Table 2. PBL strategy teaching experimental research design model
|
Research Instrument
In this study, the findings presented quantitative and qualitative data and explored students’ creativity in their learning through surveying equipment and data collection. The evaluation tools, the issues-based situational LPQ, included the personal background of participants and a structured learning attitude questionnaire of a five-point Likert scale, with options such as strongly agree, agree, neutral, disagree, and strongly disagree. The background information given by the students served as independent variables in the study. Furthermore, LPQ’s three subscales provided dependent variables.
The draft concerning the questionnaire (Su, 2022) invited three experts to conduct a substantive review, revision, and deletion of questions. The revised questionnaire was pre-tested after the mid-term exam in the second semester of the 2021 academic year. A total of 44 students participated in the pre-test. After factor analyses for Kaiser-Meyer-Olkin data (0.686) and χ2 data (882,588,928) of Bartlett spherical study showed significant initial results, factor analyses were considered appropriate for the questionnaire. The primary component analyses of the questionnaire took three factors into account. Three initial Eigenvalues were over 1.0 with an accumulative explanatory variation of 88.23%. The three aspects’ eigenvalues were 1.331, 3.094, and 10.573. All factor analysis results obtained three dominant dependent variables of the questionnaire: Qa (course satisfaction), Qb (classroom learning environment), and Qc (learning effectiveness). Then, using SPSS to analyse three variables. The questionnaire has 17 test questions and their loading factors in Appendix A. The overall Cronbach’s alpha reliability value was .946, and it became a formal questionnaire. According to the pre-test, the overall questionnaire mean (M) is 4.000, standard deviation (SD) is .688, and Cronbach’s alpha value is more than .9. According to the literature (Salta & Tzougraki, 2004; Su, 22), the reliability of any scale whose coefficient reaches .90 or above indicates that the internal consistency reliability of the scale presents an excellent level.
Data Collection & Ethics
This study focuses on quantitative data collection and descriptive statistical analysis. Conduct computer English coding and data review on the data collected before and after the experimental teaching. Statistical methods included Cronbach’s alpha internal consistency of test questionnaires, descriptive statistical analysis, and one-way ANOVA. SPSS 25.0 statistical analysis program for MS Windows. The experimental teaching conducted in this study was conducted with the informed consent of the students and complied with ethical considerations. All students are entirely based on volunteers and active participation to conduct this research. Students also agreed to participate in this study.
RESEARCH RESULTS
Teaching Material Design
The teacher plays the role of facilitator and guide in the teaching material design through the constructive learning theory of Ausubel (2000).
Designing authentic AI social application scenario textbooks in life: scenario 1, Facial recognition systems; scenario 2, smart streetlights and drone intelligence. Integrate two-scenario issues into PBL teaching to guide student group interaction and discussion, allowing students to ponder over and over again and strengthen the conceptual profound understanding. In the facial recognition system, students encounter two ill-structured problems. One is “does AI pedestrian trajectory interfere with other people’s freedom or privacy?” Another is “will facial recognition systems increase or reduce crime?” However, streetlights and drones also encounter two ill-structured problems, such as “does poor message reception contribute to crime rates?” and “can the detection accuracy of smart drones reach 100%?” Students move from encountering ill-structured problems to problem-solving planning, using self-research and data collection, and starting from group discussions. They establish problem-solving consensus and propose appropriate solutions to complete problem-solving tasks.
Descriptive Statistical Analysis
After the experimental teaching, all students engaged in the test of the structured questionnaire. The descriptive statistical analysis of their situational issues’ effective recovery rate was 100%. There were three aspects (Qa, Qb, and Qc) in LPQ and described, as follows:
Course satisfaction (Qa)
72.90% of students thought that the teacher’s teaching methods were helpful for their learning in this course. 62.50% of students pointed out that group reports and discussions were meaningful for their learning integration in this course. 62.50% of students expressed satisfaction with the course content and schedule. 68.80% of students thought that PBL course is helpful for future self-learning. And 68.80% of students were satisfied with the overall course of this semester.
Learning situation in classroom (Qb)
A fundamental feature of this course is their problem-based live learning setting. The issue included asking questions, cooperating with learning, diverse information, building cohesion and emotional support, and challenging, attracting, and inspiring my learning. Table 3 shows the degree of agreement with the learning situation. This agreement in Table 3 is more than 60.00%.
Table 3. Students learning situation in this course
|
Learning effectiveness (Qc)
The average percentage of post-test is 68.70% for students’ learning effectiveness in this course, as shown in Table 4. In Table 4, the learning effectiveness of AI in natural sciences indicates to expand their professional knowledge and abilities, improve problem-solving skills, enhance analysis/integration skills, improve critical thinking skills, and implement teamwork.
Table 4. Students learning effectiveness in this course
|
DISCUSSION
The course satisfaction (Qa) analysis found that most students were satisfied with the course learning, and more than 2/3 of the students believed that PBL course would be helpful for future self-learning. It indicated that student learning satisfaction presented positive attributes. This result echoed scholars (Adesope & Nesbit, 2012; Lin & Atkinson, 2011; Su, 2014) who found that PBL-guided learning could help improve students’ satisfaction with PBL learning (Adesope & Nesbit, 2012).
The dimensional analysis of students’ learning situations (Qb) found that students in this course actively share opinions and speak, participate in group discussions, and cooperate and interact with classmates. They used diverse information to help complete various group discussion topics and build cohesion and affection with classmates. Support, the course is challenging, and I could learn new things. They feel it will entice me even though some learning in this research may be complicated. They put a lot of learning effort into this course. Most students agree with this learning situation. During PBL learning process, students could actively participate in group discussions. 60.40% of students feel that even challenging subjects will appeal to them.
Scholars (Alan et al., 2019; Mohtar et al., 2019) emphasized that integrating cross-discipline learning from different disciplines could help improve students’ self-efficacy, ensure sustained learning interest, and produce better creations.
Therefore, the results of this study echoed the arguments of scholars. To combine the content of natural science courses, such as facial recognition systems, streetlights, and drones, its cognitive and visual experience used emerging technology products to leverage hands-on and brain-based diverse cross-disciplinary. The strategies integrated learning to improve students’ problem-solving abilities to apply knowledge and present their scientific and technological literacy with high-order scientific thinking.
In terms of students’ evaluation of the learning effectiveness of this course (Qc), most students agreed that this course could expand their professional knowledge and abilities, improve their problem-solving skills, strengthen their analysis and integration skills, and enhance their critical thinking and profound understanding. Furthermore, to demonstrate collaboration implementation and approaches. By incorporating PBL teaching into AI courses, most students have improved their problem-solving abilities, expanded their professional knowledge, and completed tasks due to group teamwork. Just like the research by Su (2021a), it revealed that the purpose of promoting educational communication technology research was to enable students to evolve from knowledge transfer to application of knowledge. In summary, from the quantitative analysis of three aspects, this teaching strategy improves students’ course satisfaction, situational learning and learning effectiveness.
CONCLUSIONS & IMPLICATIONS
Conclusions
The successful outcomes of integrating AI emerging technologies into natural science education and PBL teaching approach were encouraging and based on the findings and discussions in this research. After the second thematic lecture on “PBL~problems and problem-solving” and “application of AI in daily life” and AI life situation PBL, students have a profound understanding of facial recognition systems, streetlights, and drones.
They produced a self-scaffolding to solve problems in natural science courses by AI in life. Students could use the scaffolding successfully to solve the technological and social issues in life. However, they should improve their thinking skills in facing dilemmas and learn to be diverse. Exploring thinking and relationships, problem-solving abilities, and understanding the principle of “education is life,” as stated by American educational psychologist Dewey (1915) in (Su, 2021b).
This research summarized three conclusions, as follows:
-
Most students are satisfied with AI in PBL teaching.
-
Most students believe that cross-disciplinary learning from different disciplines could help improve self-learning efficacy and ensure sustained interest.
-
Many students agreed that this course could improve their learning effectiveness in the questionnaire.
Problems Encounters
The problems encountered during the implementation of the course were, as follows:
-
Students need to spend time strengthening the concepts for PBL building. PBL course is novel for some students. Therefore, arrange lectures from professional teachers so that students can improve the way of conducting PBL courses. However, a few students have low attendance and often feel overwhelmed during group discussions. This dilemma requires constant reminders from classroom teachers. Especially during the COVID-19 pandemic, online group presentations are so arduous.
-
The cognitive level of AI courses puts pressure on a small number of learners. Even though the application knowledge of AI in life has become more and more extensive, students are still confused about the solution structure of the problems faced by facial recognition systems, streetlights, and drones in daily life. Even though the Author invites experts to lecture, they are still confused. Some students’ understanding of new knowledge needs strength, even though some courses are laborious. It shows the students’ resilience and eagerness to complete the learning tasks of this PBL course.
Implications
The above data revealed that their logical reasoning and thinking capabilities may help them solve problems. A descriptive statistical investigation of learning perceptions demonstrated that cooperative learning improves their positive thinking abilities. In addition to comparing, evaluating, and reasoning with literature, students learned issues that needed to be answered quickly in science class and then practiced the educational objectives. As a result, from the standpoint of teaching practice and future research on design thinking, the following two recommendations were made:
-
Suggestions for teaching practice incorporate easy-to-use AI teaching materials into teaching practice and construct immersive experiential software design to improve the effectiveness of students’ experiential learning. As a result, schools should build professional classrooms and acquire the required software. Hardware enhances interaction between students and emerging technologies, embraces emerging technologies, and is at the forefront.
-
Suggestions for follow-up teaching research design. Due to the limited number of samples studied in this research, when making inferences carefully. We hope that more effective data can be added in the future, thereby increasing depth and breadth of experiment.
Funding: No funding source is reported for this study.
Acknowledgements: The author would like to thank Ministry of Education’s 2022 HDUT higher education deepening plan in Taiwan.
Ethical statement: The author stated that experimental teaching conducted in this study was conducted with informed consent of students & complied with ethical considerations. The author further stated that the highest ethical practices were followed during the study.
Declaration of interest: No conflict of interest is declared by the author.
Data sharing statement: Data supporting the findings and conclusions are available upon request from the author.
APPENDIX A
Factor Loadings of learning perception questionnaire are shown in Table A1.
Table A1. Factor Loadings of learning perception questionnaire
|
References
- Adesope, O. O., & Nesbit, J. C. (2012). Verbal redundancy in multimedia learning environments: A meta-analysis. Journal of Educational Psychology, 104(1), 250-263. https://doi.org/10.1037/a0026147
- Alan, B., Zengin, F. K., & Kececi, G. K. (2019). Using STEM applications for supporting integrated teaching knowledge of pre-service science teachers. Journal of Baltic Science Education, 18, 157-170. https://doi.org/10.33225/jbse/19.18.158
- Alimisis, D. (2013). Educational robotics: Open questions and new challenges. Themes in Science & Technology Education, 6(1), 63-71.
- Ausubel, D. P. (2000). The acquisition and retention of knowledge: A cognitive view. Kluwer Academic Publishers. https://doi.org/10.1007/978-94-015-9454-7
- Bansal, P., Kockelman, K. M., & Singh, A. (2016). Assessing public opinions of and interest in new vehicle technologies: An Austin perspective. Transportation Research Part C: Emerging Technologies, 67, 1-14. https://doi.org/10.1016/j.trc.2016.01.019
- Benitti, F. B. V. (2012). Exploring the educational potential of robotics in schools: A systematic review. Computers & Education, 58(3), 978-988. https://doi.org/10.1016/j.compedu.2011.10.006
- Di Eugenio, B., Fossati, D., & Green, N. (2021). Intelligent support for computer science education: Pedagogy enhanced by artificial intelligence. CRC Press. https://doi.org/10.1201/9781315168067
- Gadanidis, G. (2017). Artificial intelligence, computational thinking, and mathematics education. The International Journal of Information and Learning Technology, 34(2), 133-139. https://doi.org/10.1108/IJILT-09-2016-0048
- Gunter, T., & Alpat, S. K. (2017). The effects of problem-based learning (PBL) on the academic achievement of students studying ‘electrochemistry.’ Chemistry Education Research and Practice, 18(1), 78-98. https://doi.org/10.1039/C6RP00176A
- Halim, M., & Saat, R. M. (2017). Exploring students’ understanding in making a decision on a socioscientific issue using a persuasive graphic organizer. Journal of Baltic Science Education, 16(5), 813-824. https://doi.org/10.33225/jbse/17.16.813
- Hernández-Ramos, J., Pernaa, J., Caceres-Jensen, L., & Rodriguez-Becerra, J. (2021). The effects of using socio-scientific issues and technology in problem-based learning: A systematic review. Education Sciences, 11(10), 640. https://doi.org/10.3390/educsci11100640
- Hsu, C.-C., Zhuang, Y.-X., & Lee, C.-Y. (2020). Deep fake image detection based on pairwise learning. Applied. Sciences, 10, 370. https://doi.org/10.3390/app10010370
- Huang, S. P. (2018). Effects of using artificial intelligence teaching system for environmental education on environmental knowledge and attitude. EURASIA Journal of Mathematics, Science and Technology Education, 14(7), 3277-3284. https://doi.org/10.29333/ejmste/91248
- Jang, J., Jeon, J., & Jung, S. K. (2022). Development of STEM-based AI education program for sustainable improvement of elementary learners. Sustainability, 14(22), 15178. https://doi.org/10.3390/su142215178
- Jansson, S., Söderström, H., Andersson, P. L., & Nording, M. L. (2015). Implementation of problem-based learning in environmental chemistry. Journal of Chemistry Education, 92, 2080-2086. https://doi.org/10.1021/ed500970y
- Lai, C. Y. (2016). Training nursing students’ communication skills with online video peer assessment. Computers & Education, 97, 21-30. https://doi.org/10.1016/j.compedu.2016.02.017
- Lee, H., & Bae, S. (2008). Issues in implementing a structured problem-based learning strategy in a volcano unit: A case study. International Journal of Science and Mathematics Education, 6, 655-676.
- Lin, L., & Atkinson, R. K. (2011). Using animations and visual cueing to support learning of scientific concepts and processes. Computers & Education, 56, 650-658. https://doi.org/10.1016/j.compedu.2010.10.007
- Mohtar, L. E., Halim, L., Rahman, N. A., Maat, S. M., Iksan, Z. H., & Osman, K. (2019). A model of interest in STEM careers among secondary school students. Journal of Baltic Science Education, 18(3), 404-416. https://doi.org/10.33225/jbse/19.18.404
- Mundilarto, H. I. (2018). Effect of problem-based learning on improvement physics achievement and critical thinking of senior high school student. Journal of Baltic Science Education, 16(5), 761-780. https://doi.org/10.33225/jbse/17.16.761
- Ng, E. M. (2016). Fostering pre-service teachers’ self-regulated learning through self-and peer assessment of wiki projects. Computers & Education, 98, 180-191. https://doi.org/10.1016/j.compedu.2016.03.015
- Nordhoff, S., van Arem, B., Merat, N., Madigan, R., Ruhrort, L., Knie, A., & Happee, R. (2017). User acceptance of driverless shuttles running in an open and mixed traffic environment [Paper presentation]. The 12th ITS European Congress.
- Prince, M. J., & Felder, R. M. (2006). Inductive teaching and learning methods: Definitions, comparisons, and research bases. Journal of English Education, 95, 123-138. https://doi.org/10.1002/j.2168-9830.2006.tb00884.x
- Ricoy, M. C., & Feliz, T. (2016). Twitter as a learning community in higher education. Journal of Educational Technology & Society, 19(1), 237.
- Rihtaršič, D., Avsec, S., & Kocijancic, S. (2016). Experiential learning of electronics subject matter in middle school robotics courses. International Journal of Technology and Design Education, 26(2), 205-224. https://doi.org/10.1007/s10798-015-9310-7
- Rillero, P., & Chen, Y. C. (2019). The use of a digital problem-based learning module in science methods courses. Journal of Problem Based Learning in Higher Education, 7(1), 107-119. https://doi.org/10.5278/ojs.jpblhe.v7i1.2349
- Sakir, N. A. I., & Kim, J. G. (2020). Enhancing students’ learning activity and outcomes via implementation of problem-based learning. EURASIA Journal of Mathematics, Science and Technology Education, 16(12), em1925. https://doi.org/10.29333/ejmste/9344
- Salta, K., & Tzougraki, C. (2004). Attitudes toward chemistry among 11th grade students in high schools in Greece. Science Education, 88(4), 535-547. https://doi.org/10.1002/sce.10134
- Sevian, H., Bernholt, S., Szteinberg, G. A., Auguste, S., & Pérez, L. C. (2015). Use of representation mapping to capture abstraction in problem solving in different courses in chemistry. Chemistry Education Research and Practice, 16(3), 429-446. https://doi.org/10.1039/C5RP00030K
- Su, K. D. (2014). Integrated molecular chemistry teaching on 12th grade students’ learning performance. Research of Educational Communications and Technology, 107, 1-10.
- Su, K. D. (2018). Enhancing students’ corresponding reasoning of cognitive performances by animated concept mapping in electrochemistry. Journal of Baltic Science Education, 17(4), 662-673. https://doi.org/10.33225/jbse/18.17.662
- Su, K. D. (2021a). A new assessment of HOCS-oriented learning for students’ higher-order thinking abilities by Marzano’s taxonomy. Journal of Baltic Science Education, 20(2), 305-315. https://doi.org/10.33225/jbse/21.20.305
- Su, K. D. (2021b). Technology and Living (6thEd.).New Wun ChingDevelopmental PublishingCo.
- Su, K. D. (2022). Implementation of innovative artificial intelligence cognitions with problem-based learning guided tasks to enhance students’ performance in science. Journal of Baltic Science Education, 21(2), 245-257. https://doi.org/10.33225/jbse/22.21.245
- Sullivan, F. R., & Heffernan, J. (2016). Robotic construction kits as computational manipulatives for learning in the STEM disciplines. Journal of Research on Technology in Education, 48(2), 105-128. https://doi.org/10.1080/15391523.2016.1146563
- Syadiyah, A. S., Mohammad, N. N., & Azrul, A. I. M. (2017). Integration of naqli and aqli elements in problem based learning (PBL). Education Journal, 6(6), 164-169. https://doi.org/10.11648/j.edu.20170606.11
- Topcu, M. S., Sadler, T. D., & Yilmaz-Tuzun, O. (2010). Preservice science teachers’ informal reasoning about socioscientific issues: The influence of issue context. International Journal of Science Education, 32(18), 2475-2495. https://doi.org/10.1080/09500690903524779
- Yoon, H., Woo, A. J., Treagust, D., & Chandrasegaran, A. L. (2014). The efficacy of problem-based learning in an analytical laboratory course for pre-service chemistry teachers. International Journal of Science Education, 36(1), 79-102. https://doi.org/10.1080/09500693.2012.727041
- Zulić, H. (2019). How AI can change/improve/influence music com¬position, performance and education: Three case studies. INSAM Journal of Contemporary Music, Art and Technology, 1(2), 100-114. https://doi.org/10.51191/issn.2637-1898.2019.2.2.100
How to cite this article
APA
Su, K.-D. (2024). Problem-based life situational issues exploration–Taking the learning effectiveness of artificial intelligence in natural sciences. Interdisciplinary Journal of Environmental and Science Education, 20(2), e2406. https://doi.org/10.29333/ijese/14420
Vancouver
Su KD. Problem-based life situational issues exploration–Taking the learning effectiveness of artificial intelligence in natural sciences. INTERDISCIP J ENV SCI ED. 2024;20(2):e2406. https://doi.org/10.29333/ijese/14420
AMA
Su KD. Problem-based life situational issues exploration–Taking the learning effectiveness of artificial intelligence in natural sciences. INTERDISCIP J ENV SCI ED. 2024;20(2), e2406. https://doi.org/10.29333/ijese/14420
Chicago
Su, King-Dow. "Problem-based life situational issues exploration–Taking the learning effectiveness of artificial intelligence in natural sciences". Interdisciplinary Journal of Environmental and Science Education 2024 20 no. 2 (2024): e2406. https://doi.org/10.29333/ijese/14420
Harvard
Su, K.-D. (2024). Problem-based life situational issues exploration–Taking the learning effectiveness of artificial intelligence in natural sciences. Interdisciplinary Journal of Environmental and Science Education, 20(2), e2406. https://doi.org/10.29333/ijese/14420
MLA
Su, King-Dow "Problem-based life situational issues exploration–Taking the learning effectiveness of artificial intelligence in natural sciences". Interdisciplinary Journal of Environmental and Science Education, vol. 20, no. 2, 2024, e2406. https://doi.org/10.29333/ijese/14420